From container shipping to artificial intelligence, demand forecasting ensures ‘just right’ service
Think about your last flight. There probably weren’t a lot of empty seats. And at the grocery store, where we’re again seeing store shelves with the just-right amount of stock, it’s troubling when some products are completely out. That’s a sign that there's a problem in the pipeline, something that demand forecasting tries to protect against happening.
Demand forecasting is a process for how inventories and assets that are needed to move them are planned and sustained. The process uses artificial intelligence (AI) and machine learning to analyze historical data, including buying patterns. The technologies help industries to estimate customer demand for a product, like store shelf items, or service, like air travel, so there’s no glut or shortage.
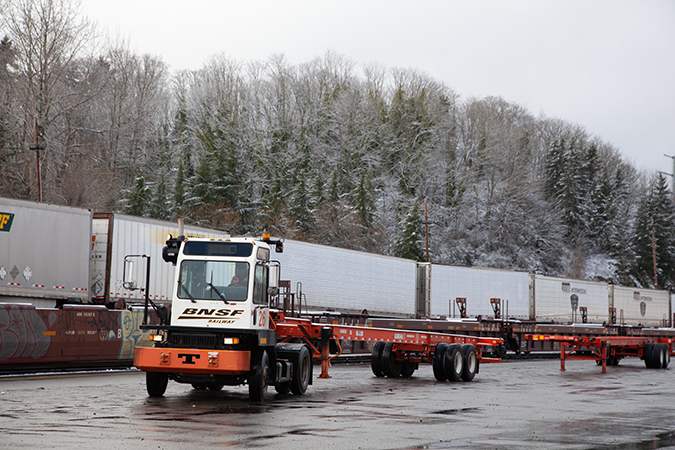
To make sure our customers’ just-right amounts of goods and materials are received when they expect and need them, we’re also using demand forecasting models that rely on AI. In our intermodal business, which is focused on consumer goods, having a precise demand forecasting model helps us have just-right resources – like locomotives, railcars, cranes, hostlers, and people – available, plus the service plans in place to move trains.
“Like meteorologists predicting the weather, getting the just-right forecast depends on the model,” said April Kuo, director of intermodal analytics at BNSF. “These models rely on internal data, including historical patterns, as well as external data from customers and others in the supply chain.”
At a railroad terminal or intermodal hub, capacity is fixed, so having a reliable model that predicts upcoming in-gate volumes of containers and trailers seven days out will help us better manage inventory in a hub. The model will help us see when there’s potential congestion so local teams can plan ways to mitigate, Kuo added.
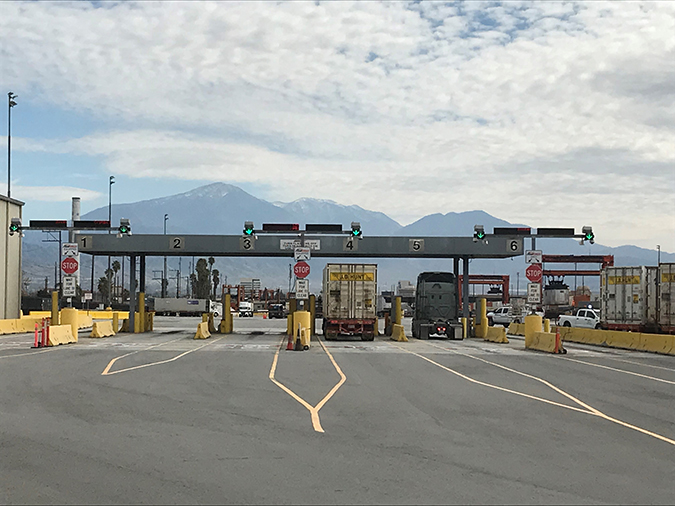
Based on the forecast, we will plan for when a unit (container or trailer) starts its intermodal journey, beginning with when it enters a BNSF intermodal hub. From there, the unit goes to a designated parking spot to wait for its planned outbound train until a hostler moves it trackside so a crane can load it onto a train. The train takes it to the destination hub, and a customer will be notified to pick it up.
For each given unit, the entire rail shipping process involves many decisions equal to 1 million combinations of choices. With more than 5 million units handled through BNSF’s intermodal network per year, the amount of data we collect enables us to develop AI that can be used to standardize, automate and optimize as many decisions across the intermodal value chain as possible.
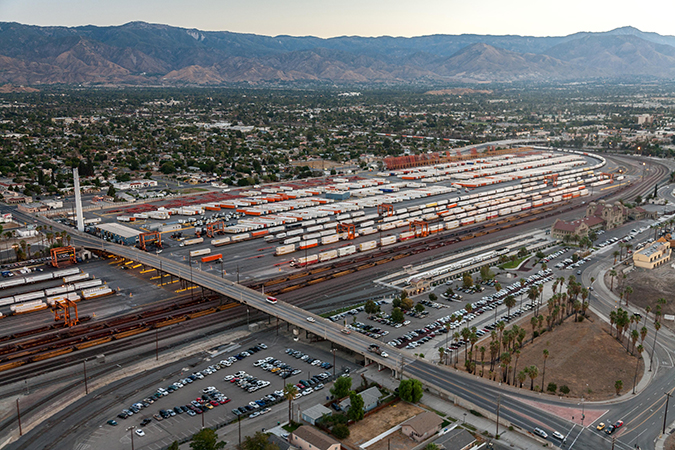
The demand forecast model will also support other technologies, which is why Kuo and her team will test and validate the model before fully implementing it. “Like the weather, even just a few degrees off from the forecast can have a significant impact,” she noted.
The demand forecast model is just one way we’re using AI at BNSF as a foundation to improve business processes. It will feed other AI technologies to optimize our existing infrastructure and equipment, which will improve efficiency, capacity utilization, customer experience and consistency.
Forecasting using AI is also being developed to support the recent addition of Quantum, a J.B. Hunt and BNSF service, which accommodates customers’ service-sensitive highway freight. The technology will aid Quantum service at every step of the intermodal shipping process, from planning to execution and oversight to exception management. AI integration allows the Quantum team to automatically identify variability and enable the team to quickly detect and resolve issues before they affect final delivery.
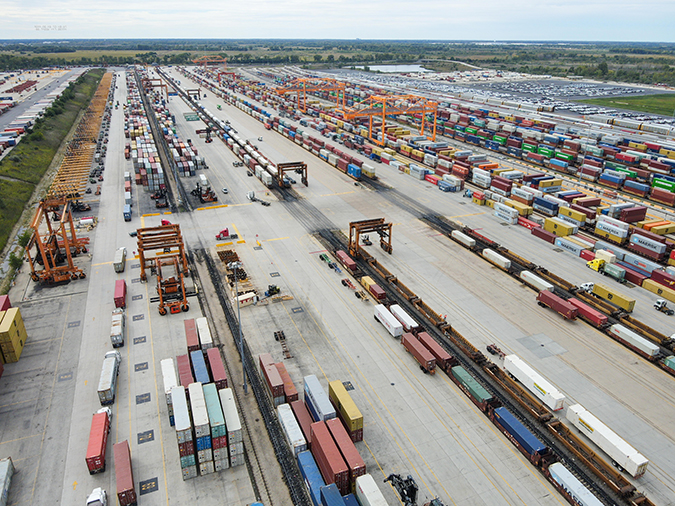
“Starting with our intermodal business and eventually cascading to our other businesses, we see many opportunities for using AI,” said Jon Gabriel, vice president of Service Design. “The endgame is to reduce variability, which leads to more consistent and reliable service.”