Artificial intelligence: A new frontier for safety, efficiency and service at BNSF
Artificial intelligence (or AI) has been a big topic in the news lately, with the rising popularity of AI software like ChatGPT and Midjourney. AI also has many industrial and commercial applications. The rapidly evolving technology is transforming how information is processed, analyzed and used to support sound decision-making.
BNSF Railway has always been at the forefront of leveraging cutting-edge technologies for the right reason, and AI is no different. AI has the potential to help us make significant improvements in safety, asset utilization, service and operational efficiency.
From a safety perspective, thanks to machine vision technology, sensors on locomotives, wayside detectors and other systems, we have vast troves of data that AI tools can work with to predict potential defects of cars, locomotives or rail and to help prevent major failures. We’re also using AI to improve our business processes for shipping efficiency and accuracy as well as to supplement capacity.
“We have two ways to add capacity. We can physically expand the capacity of our network, including adding new or expanding facilities, as well as by adding new tracks, new parking lots or equipment such as hostlers and cranes,” April Kuo, director, Network Strategy, Design & Innovation, said. “But a real emerging opportunity to add capacity while improving service is optimizing the way we use our existing infrastructure and equipment by leveraging AI to change business processes to improve efficiency.”
While this technology is helping us to enhance and standardize processes, we also rely on our subject matter experts – humans – to share their experience and lean on their business decisions.
Here’s a look at some of the projects and the technologies that BNSF has engineered that will transform how we serve customers in the future:
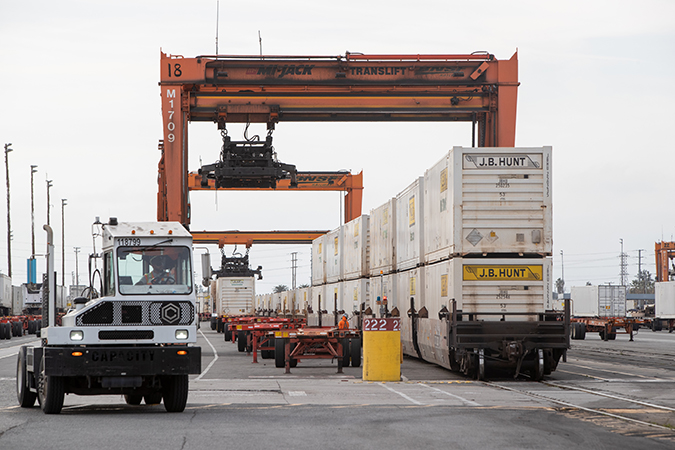
Hostler Next-Best Move
At our intermodal hubs, where containers and trailers arrive or depart by train or truck and are transferred between modes, we’ll soon expand our testing of AI to enable hostler drivers to move the units more efficiently from parking lots to tracks or from tracks to parking lots.
Currently, in an intermodal facility, hostler drivers rely on manual instruction by radio from ramp supervisors. For example, to build an outbound train, they work as a team to move the units from various parking lots to a parking lane next to the track, called production tracks. The drivers spot (position) the units trackside so cranes can lift the units onto the railcar in a particular sequence, maximizing crane utilization.
Which units are taken by which drivers is manually assigned by a ramp supervisor. The drivers’ route is somewhat random, meaning they might be driving to the farthest ends of a parking lot to retrieve a unit, then repeating the process over and over.
With cloud computing and asset digitalization that factors in tracks, railcars, units, hostlers and cranes through AI, the new technology produces a dynamic algorithmic assignment plan. The plan tells the drivers in real time where the nearest units are to them, optimizing the distance and time it takes to load a railcar. Considering that there may be as many as 10 hostlers building one outbound train, the benefits add up.
These new algorithms are being tested at our Alliance, Texas, intermodal facility. This technology will reduce hostler driving distance, carbon emissions and the time it takes to load and unload. And by automating the repetitive nature of hostlers’ work, they will now be more efficient and able to handle issues sooner.
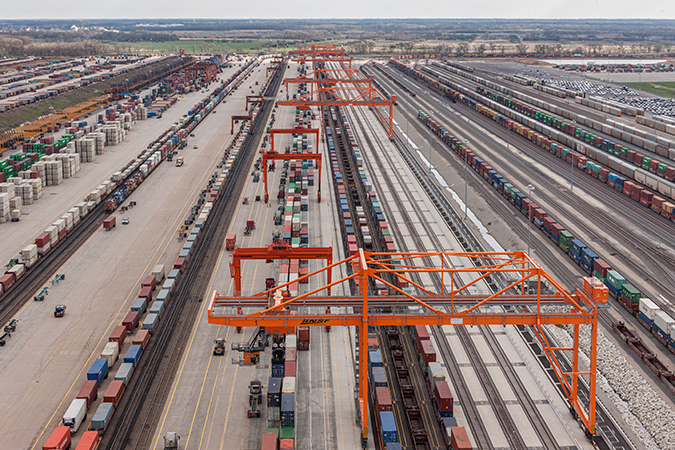
Load Planning Optimization
In addition to AI improving how hostlers get containers and trailers spotted trackside more efficiently at hubs when building outbound trains, we’re taking another major step forward: optimizing how we load each railcar with a container or trailer.
Traditionally, the process for planning how an outbound train is loaded (around 200 or more units) is manual and must follow load-planning rules that account for the weight of the container, its length and, in some cases, what’s inside. The plan also must ensure that if a well car is double stacked, the containers are stacked in the proper configuration. Should a unit be improperly assigned according to the rules, time is lost while the error is corrected.
Using an AI-based algorithm that creates in seconds a load plan for an outbound train, we can ensure that the right containers and trailers are located where they need to be on the production tracks – all according to the plan and minimizing the overall distance by hostler drivers. By better planning which container or trailer goes on which car, and in the case of double-stacked containers, whether they should go on bottom or top, we save valuable loading time. We also have better slot utilization, meaning all available space on an outbound train is utilized.
With the new algorithms tested at our Alliance, Texas, hub, we improved the time it took to load the train on average by more than 30 minutes. With about 10 outbound trains a day, turning that production track faster gives us added capacity of about 30,000 additional lifts annually. We plan to begin scaling this solution across the network and anticipate increasing total capacity to 500,000 lifts annually just from this one improvement.
Additionally at Alliance, hostlers’ driving distances have decreased an average of 20 miles per train loaded – improving fuel consumption and enhancing sustainability.
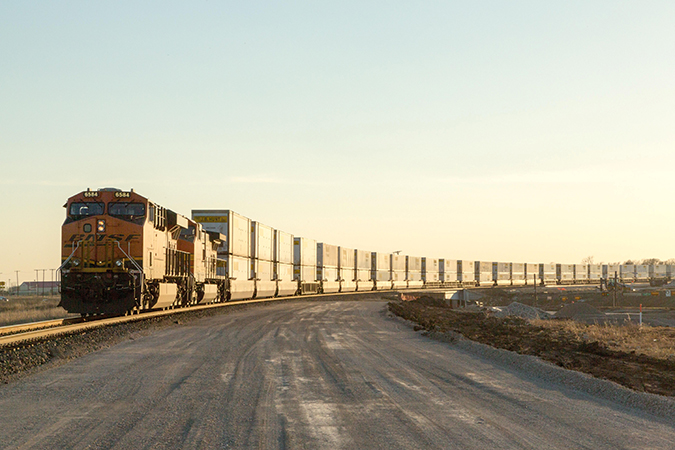
Intermodal Train Estimated Times of Arrival
Another project for our intermodal operations uses algorithms to predict the length of time an intermodal train will be at the terminals or stations along its route.
Between origination and destination, an intermodal train will go through numerous terminals or stations. For example, a train departing Chicago for Southern California will pass through nine terminals before reaching its destination. When an intermodal train enters a terminal, it will need to get a new crew and may need to be refueled and/or inspected, all of which takes time – what we call "dwell."
The dwell time may also be increased by the number of trains currently in the terminal and the amount of work associated with each train. Our transportation system plans for these dwell times, which are unavoidable – but sometimes plans and reality vary.
The AI algorithm now being tested uses the recent history of similar trains as they go through each terminal over the course of their route and better predicts dwell time. The result, based on short- and long-term history, is that we can add up the dwell times and more consistently predict when the train will arrive at its destination.
Early testing found this new dwell methodology improved one type of intermodal train’s ETA by as much as 20%, helping both customers and our operating teams better plan their day.
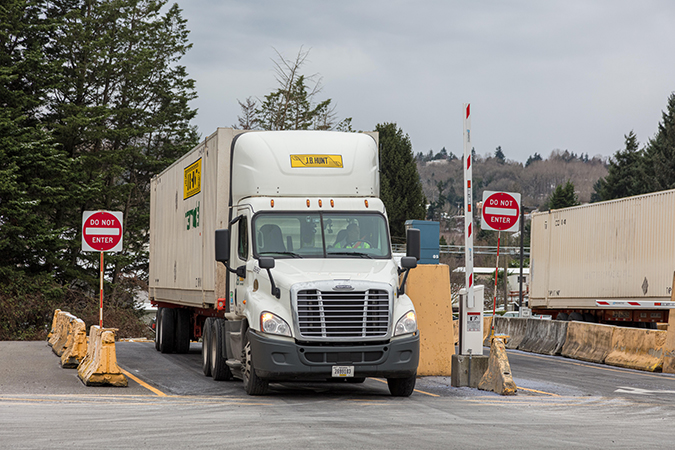
In-Gate Forecasting
Just like a warehouse manager needs to know how much inventory is coming and going, at our intermodal hubs, we need to know how many containers and trailers we can expect to receive to keep the facility fluid.
When we don’t know how many units a hub will receive every day in advance, it affects the hub’s ability to provide the best service plan for customers. For example, when a hub’s inventory is approaching capacity, an outside driver dropping off a unit may park it randomly or double-park it, creating an obstacle that not only slows operations but makes it harder to keep accurate track of inventory. If we can forecast the days when inventory level is going to be high, hubs can better respond to the potential surge.
Using machine learning to look back at historical ebbs and flows at a hub over the past four years, we’ll be able to better predict how many units will be received by our facilities as far as 10 days out. By being able to forecast farther out and communicate with customers, we’ll take a lot of the guesswork out of the process. In addition, accurate in-gate forecasts help us better prepare our transportation service plan and improve slot utilization for each train.
Having customer-centric information, as well as the experience of our hub teams, will build in flexibility – and be foundational for many more projects going forward.
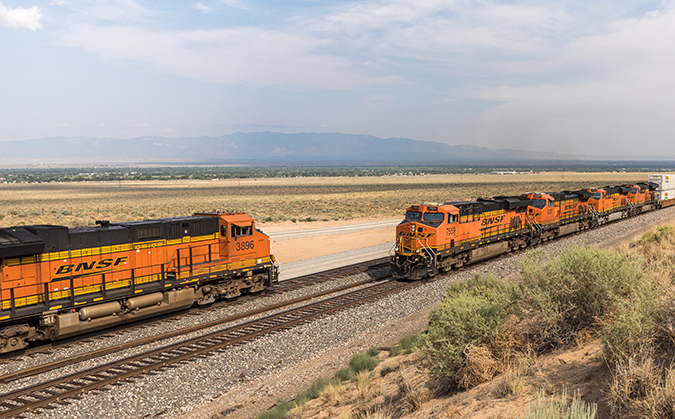
Traffic Volume, Velocity, Density
Most drivers use a technology very similar to this as they plan their commute or trip, with mobile apps that show what the roadway situation looks like ahead: Red indicates dense traffic, yellow is moderate density and green means traffic is flowing smoothly.
At the railroad, there’s no technology that reports real-time traffic flow that encompasses volume, density and velocity – only operating condition scorecards that tell our teams what the traffic levels are based on the volume of trains. It doesn’t account for the velocity of those trains to give us a clear picture of what our capacity opportunities and potential bottlenecks are.
We’re testing an industry-leading solution that will help us stay ahead of the curve and be less reactive. Real-time data will help us look at conditions across our network and predict up to 72 hours out where we may have congestion so we can address the issue before it occurs.
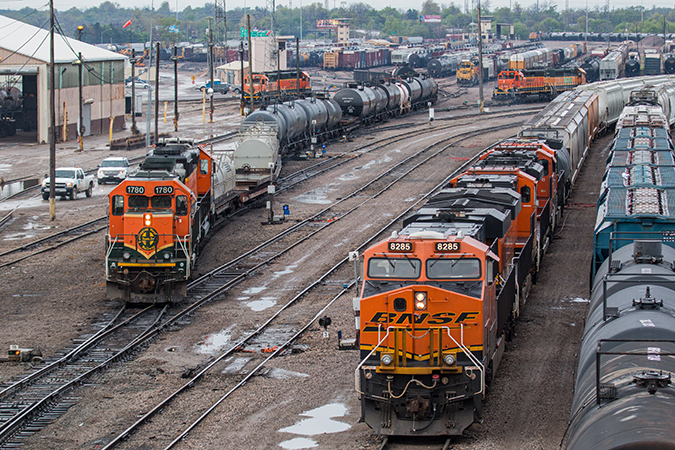
Switch List Optimization for Merchandise Flat Yards
In a merchandise flat yard, we assemble and disassemble trains to build new ones with freight sorted for different destinations. The process of picking and sorting freight cars on these trains is done by switch crews using locomotives to push and pull the cars, following a switch list that has instructions that tell the crew on which track to place the cars.
Within a yard, there may be dozens if not hundreds of tracks, switches and signals, making the process even more time- and labor-intensive. For example, imagine 100 freight cars are on one track and 100 cars on another. The outbound train being built needs 50 cars from each track. AI can help solve the puzzle and make the switching process to build that new train as efficient as possible.
Using historical data to know how many cars customers are providing, where they are coming from and where they are going, AI will help us to receive and build those cars in a sequence – blocks instead of individual cars – to minimize the time it takes to move the cars into position. We’re researching how AI can optimize the sequencing and processing of blocks of cars to minimize the number of moves our employees must make.
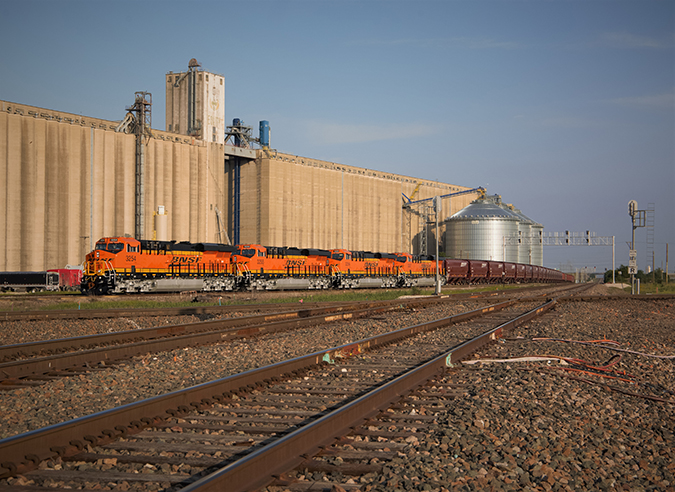
Grain Train Estimated Time of Arrival
Another AI initiative aims to improve estimated arrival times for our grain shuttle train customers.
Grain shuttles are non-stop high-volume dedicated freight trains, usually hopper cars, filled with single-bulk commodities, like corn, soybean or wheat. The trains are usually made up of 110 cars that cycle between an origin point for loading (a grain elevator) and destination for unloading (a facility or port).
These customers want an arrival window of three to four hours – just like when you order from Amazon, you get a timestamp for delivery by day and timeframe. By having that window, our customers can make better decisions about when to have crews on site. Better information not only helps them make better decisions, it also builds trust in our service.
We’re identifying data points that affect this service so we can help improve customer train loading operations and reduce the time it takes get the train moving toward destination.
“AI has the potential to deliver huge benefits in a broad range of areas, including safety, efficiency, velocity and consistency,” Jon Gabriel, vice president, Service Design, said. “It’s an exciting time for us as we leverage this technology and the data it provides to helps us continuously improve our service and capacity available for our customers.”